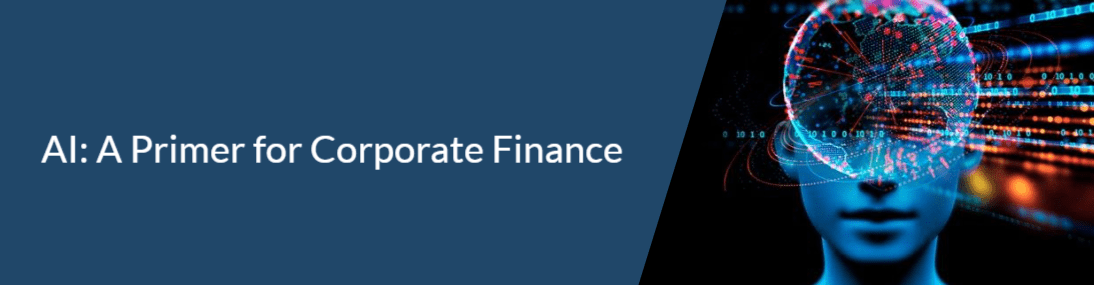
Just a few years ago, artificial intelligence (AI) was a brand new technology that stoked excitement and fear. Concerns of a terminator-induced armageddon were just as common as predictions of stunning new technological breakthroughs.
Since then, AI has transformed from frontier technology to practical business tool. While many are aware of its well-publicized feats, such as beating humans at chess and solving the protein-folding problem, AI now has much more practical uses. It can take on practical problems like natural language processing (NLP), demand forecasting, and analysis of large datasets.
CFOs are taking notice. According to a Deloitte survey, 97% of CFOs plan to employ AI in the near future, but only 2/3rds currently use it. In finance departments today, this technology is mostly deployed in the back office to detect anomalies during audits and automate repetitive processes.
But the back office is just the beginning.
Once companies have a good handle on AI, they can start to feed it data to generate predictive analytics. Predicting how consumers are likely to respond to a new product, which debts are unlikely to be paid, and where risks are likely to emerge will be a game-changer.
In short, AI’s time has come.
Starting out small
Like with any new tech, it pays to walk before you run. PWC found in its recent study that CFOs who set clear priorities, work on governance structures with employees, and start small tend to have the best results.
A typical first step is to get a handle on large datasets. Machine learning (ML) is a subset of AI that’s relatively easy to implement, and can recognize repeating patterns in data. For instance, mobile payments company Square uses ML to better detect fraud at its terminals, building upon thousands of data points to improve its recognition of hostile actors.
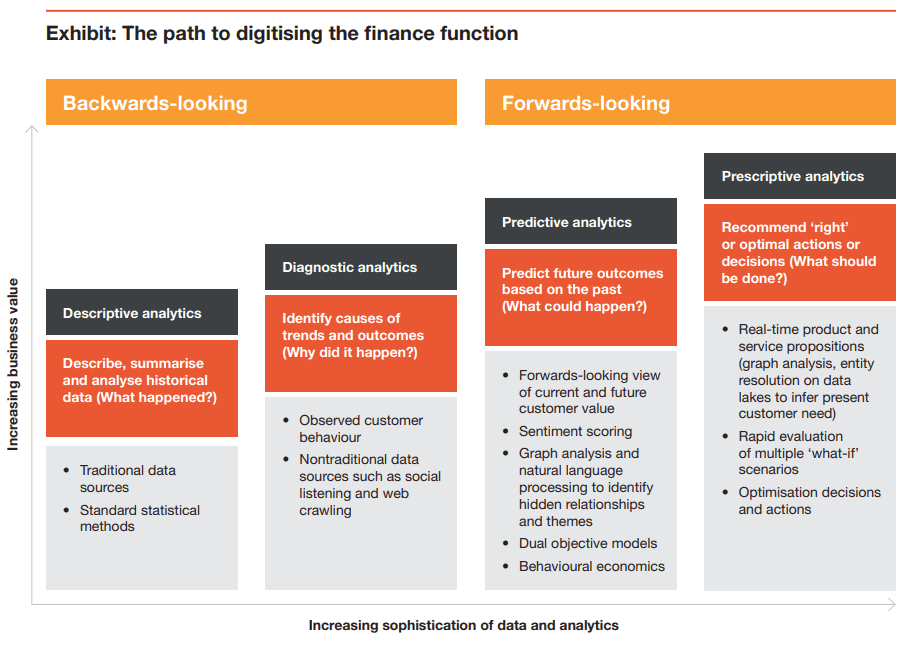
Source: PWC
Once ML apps are running smoothly and generating useful results, the next rung of the ladder opens up. Instead of simply identifying past trends, more advanced AI can start to explain why those trends emerged in the first place. For example, manufacturers have leveraged AI capabilities to respond swiftly to the wide spikes in demand since COVID.
The pinnacle of AI in corporate finance is its predictive power to answer a few key questions—such as what’s likely to happen in the future, and how can my organization best prepare for it?
Leverage multiple datasets to answer questions about the future
Prescriptive and predictive analytics sit at the top of the AI food chain. Being able to model what might happen (prescriptive), then determine how to make a desired outcome actually take place (predictive) are the biggest value-adds from AI at this point.
In finance, identifying the most impactful KPIs and figuring out how to meet them is imperative. AI can scan historical data to see how likely KPIs are to be met, then suggest optimal courses of action for getting there.
Let’s say you’re a personal protective equipment (PPE) manufacturer projecting future sales. After collating past sales data and feeding it through a predictive analytics model, you see that sales are projected to stay flat for the next five years.
Armed with that data, you can then turn to prescriptive analytics to see how you can improve that growth rate. The AI model leverages internal and external data to recommend several changes, including a product change to reflect changing consumer tastes. This is enabled by customer survey data, A/B tests, and market research.
The key enabler to these advanced functions is “clean,” relevant data. Unstructured data requires a significant amount of time to clean and stage for analysis, leaving less time for generating insights.
Consolidating Opex, Capex, market research, and other data within your company gives AI the best opportunity to make useful predictions. But this doesn’t mean your ERP and all other apps in your finance stack need AI capabilities of their own.
Rather, by collecting data across all these systems, an AI solution can leverage the combined dataset. For example, Finario Predict can help you make better capital planning decisions by using historical project data to forecast likely outcomes of future projects.
Walking before you run with AI doesn’t just help increase adoption at companies. It also ensures that they develop good data governance to enable more advanced AI capabilities such as robotic process automation (RPA).
Uses for AI in corporate finance departments today
Essentially, with RPA, a company first identifies a workflow that sets up well for automation. Then, with the help of solutions specializing in RPA, it sets up a robot to run the process. Once the bot is working well for a single process, additional tasks can be added.
Take Deutsche Bahn, for example. As the national railway company of Germany and one of the biggest transportation companies in the world, it serves over 2 billion passengers per year. That means rows upon rows of incident reports that need to be collated across applications and processed by the corporate team.
Employees were spending a significant portion of their time on the repetitive tasks of data entry and synchronizing data across systems. RPA company Cloudstorm was able to implement a robot that automatically entered relevant data and synced it across various systems. Workers reported better morale, and executives enjoyed fewer manual data entry errors.
Aiwozo is another RPA company helping businesses get started with AI. One of its success stories includes a retailer that struggled to process invoices in a timely manner, due to the variety of formats they received.
The solution to this issue was an AI program that used Optical Character Recognition (OCR) technology to convert the invoices to a standard format. The standardized data was then fed into Aiwozo’s ML model to extract relevant information, providing training data for a more advanced AI program.
The resulting bot reduced the workload for employees and generated useful insights not available before the AI solution.
Don’t get left behind
The AI revolution in corporate finance is here. Back office automations are great for eliminating tedious work, but harnessing data to make better predictions and decisions is where AI will unlock the most value.
Building a rock-solid business case is key … as is having a complete dataset. For finance, that means not just Opex and treasury, but Capex – with deep and detailed project-level data. With that single source of truth, and the capabilities of AI to leverage it, companies can create a significant competitive advantage in its operational efficiency, return on invested capital, and overall marketplace performance.